BY: ChatGPT (this article was written 100% by artificial intelligence based on input defined by Dale Cade)
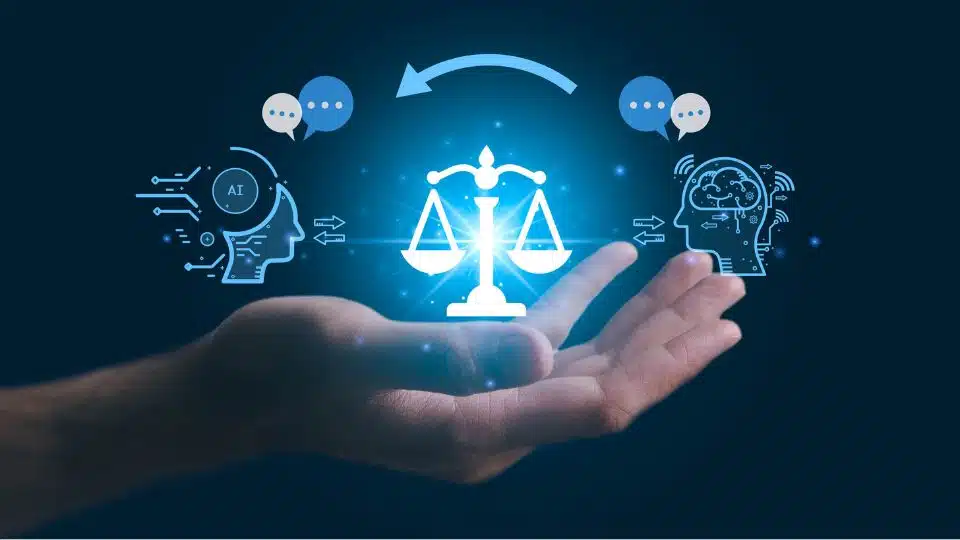
Artificial Intelligence (AI) has become an essential part of our daily lives, influencing everything from how we shop to how we receive healthcare. However, as we increasingly rely on AI for decision-making, one major concern has emerged: AI bias. In simple terms, AI bias occurs when an AI system produces results that are systematically unfair or prejudiced, often reflecting the biases present in the data it was trained on. These biases can lead to significant ethical, social, and legal implications, especially in sensitive areas such as hiring, healthcare, and criminal justice.
Understanding AI Bias
At its core, AI bias arises when an AI system makes decisions that disadvantage certain groups or individuals, often unintentionally. This happens because AI systems “learn” patterns from the data they are trained on. If this training data contains biased human decisions or reflects historical inequalities, the AI will likely perpetuate these biases.
AI systems are often viewed as objective and impartial because they are built using algorithms and vast amounts of data. However, this perception can be misleading. If the data used to train these systems is skewed or incomplete, the AI will reflect and amplify those biases, creating discriminatory outcomes.
Real-World Examples of Biased AI Systems
- Hiring Algorithms
One of the most notable examples of AI bias in the real world is in recruitment. In 2018, it was discovered that Amazon had developed an AI system to help with recruitment. The system, however, was found to be biased against women. The AI had been trained on resumes submitted to Amazon over the past 10 years, which were predominantly from male candidates. As a result, the system was biased toward resumes that included more traditionally male-associated language and experiences, such as “managing” teams or working in male-dominated fields. This example shows how bias in the training data (in this case, gender imbalance) can lead to unfair and discriminatory hiring decisions. - Predictive Policing
Another example of AI bias comes from predictive policing systems used by law enforcement. These systems analyze past crime data to predict where future crimes might occur. However, many of these systems have been criticized for disproportionately targeting minority communities. This happens because the data used to train these systems often reflects historical biases in policing practices, such as over-policing in certain neighborhoods. As a result, AI-driven predictive policing tools may lead to a cycle of over-policing in these communities, perpetuating racial disparities in the criminal justice system. - Facial Recognition Technology
Facial recognition systems have been shown to have significant bias, particularly when it comes to identifying people with darker skin tones. Studies have found that facial recognition systems are more accurate at identifying lighter-skinned individuals, while they often misidentify or fail to recognize darker-skinned individuals, especially women. In 2018, a study by the MIT Media Lab found that commercial facial recognition systems misidentified the gender of darker-skinned and female faces at a higher rate than lighter-skinned and male faces. This bias can have serious consequences in fields like law enforcement and security, where misidentification can lead to wrongful arrests or surveillance.
Why Does AI Bias Matter?
AI bias matters because the decisions made by biased AI systems can have far-reaching consequences, especially in sensitive areas where fairness, equity, and human rights are at stake. Below are some key reasons why AI bias is a critical issue:
1. Unfair Hiring Practices
As AI systems become more involved in recruitment and hiring, biased algorithms can lead to discrimination against certain groups, particularly women, racial minorities, and people with disabilities. For instance, if an AI system has been trained on biased historical data where certain groups were underrepresented, it may favor candidates from overrepresented groups, leading to fewer opportunities for underrepresented individuals. This can perpetuate inequality in the workplace, limiting diversity and stifling innovation.
2. Incorrect Business Decisions
Businesses often use AI to make data-driven decisions, such as identifying target markets, optimizing supply chains, and predicting customer preferences. If an AI system is biased, these decisions could be flawed. For example, a biased recommendation engine might suggest products based on skewed data, leading to missed opportunities in underserved markets. Similarly, biased business forecasting models could result in investments that ignore the needs of certain demographic groups, leading to lost revenue or even reputational damage.
3. Discriminatory Healthcare Decisions
Healthcare is another sector where AI bias can have particularly harmful effects. AI is increasingly being used for diagnosing diseases, predicting patient outcomes, and even recommending treatments. However, if the data used to train these systems is not representative of diverse populations, it can lead to biased healthcare decisions. For example, an AI model trained primarily on data from white male patients might misdiagnose or fail to recognize certain symptoms in women or people of color, leading to improper treatment or delayed diagnoses. This could exacerbate health disparities, particularly in underserved communities.
4. Perpetuating Social Inequality
AI systems, particularly those used in criminal justice or lending, can perpetuate existing social inequalities. For instance, AI used in risk assessments for parole decisions may be influenced by biased historical data that reflects racial discrimination in policing or sentencing. This could lead to unfairly high-risk assessments for minority individuals, leading to longer sentences or higher bail amounts, even when they are not actually a higher risk. This can reinforce cycles of inequality and disproportionately affect marginalized communities.
Conclusion
AI bias is a critical issue that requires careful attention and action from developers, regulators, and society at large. While AI systems hold the potential to drive innovation and improve decision-making, biased algorithms can have serious consequences that undermine fairness, equality, and justice. To address AI bias, it is essential to ensure that the data used to train AI models is diverse, representative, and free from historical biases. Additionally, transparency, accountability, and ethical standards must be implemented to prevent harmful outcomes. Only by addressing AI bias can we harness the full potential of artificial intelligence in a way that benefits everyone fairly.